Detecting Treatment Effects with Small Samples: The Power of Some Tests Under the Randomization Model
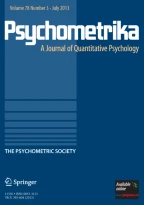
Randomization tests are often recommended when parametric assumptions may be violated because they require no distributional or random sampling assumptions in order to be valid. In addition to being exact, a randomization test may also be more powerful than its parametric counterpart. This was demonstrated in a simulation study which examined the conditional power of three nondirectional tests: the randomization t test, the Wilcoxon–Mann–Whitney (WMW) test, and the parametric t test. When the treatment effect was skewed, with degree of skewness correlated with the size of the effect, the randomization t test was systematically more powerful than the parametric t test. The relative power of the WMW test under the skewed treatment effect condition depended on the sample size ratio.
This is a preview of subscription content, log in via an institution to check access.
Access this article
Subscribe and save
Springer+ Basic
€32.70 /Month
- Get 10 units per month
- Download Article/Chapter or eBook
- 1 Unit = 1 Article or 1 Chapter
- Cancel anytime
Buy Now
Price includes VAT (France)
Instant access to the full article PDF.
Rent this article via DeepDyve
Similar content being viewed by others
Nonparametric meta-analysis for single-case research: Confidence intervals for combined effect sizes
Article 16 April 2018
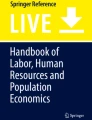
Design and Analysis of Experiments
Chapter © 2021
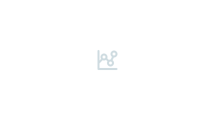
On Detecting a Minimal Important Difference among Standardized Means
Article 28 December 2016
References
- Box, G.E.P., & Anderson, S.L. (1955). Permutation theory in the derivation of robust criteria and the study of departures from assumption. Journal of the Royal Statistical Society. Series B, Statistical Methodology, 17, 1–34. Google Scholar
- David, H.A. (2008). The beginnings of randomization tests. The American Statistician, 62, 70–72. ArticleGoogle Scholar
- Dwass, M. (1957). Modified randomization tests for nonparametric hypotheses. Annals of Mathematical Statistics, 28, 181–187. ArticleGoogle Scholar
- Eden, T., & Yates, F. (1933). On the validity of Fisher’s z test when applied to an actual example of non-normal data. Journal of Agricultural Science, 23, 6–17. ArticleGoogle Scholar
- Edgington, E.S., & Ezinga, G. (1978). Randomization tests and outlier scores. The Journal of Psychology, 99, 259–262. ArticleGoogle Scholar
- Edgington, E.S., & Onghena, P. (2007). Randomization tests (4th ed.). Boca Raton: Chapman & Hall. Google Scholar
- Fisher, R.A. (1935). The design of experiments. Edinburgh: Oliver & Boyd. Google Scholar
- Gabriel, K.R., & Hall, W.J. (1983). Rerandomization inference on regression and shift effects: Computationally feasible methods. Journal of the American Statistical Association, 78, 827–836. ArticleGoogle Scholar
- Gabriel, K.R., & Hsu, C.-F. (1983). Evaluation of the power of rerandomization tests, with application to weather modification experiments. Journal of the American Statistical Association, 78, 766–775. ArticleGoogle Scholar
- Gill, P.M.W. (2007). Efficient calculation of p-values in linear-statistic permutation significance tests. Journal of Statistical Computation and Simulation, 77, 55–61. ArticleGoogle Scholar
- Hayes, A.F. (1996). Permutation test is not distribution-free: Testing H0:ρ=0. Psychological Methods, 1, 184–198. ArticleGoogle Scholar
- Hettmansperger, T.P. (1984). Statistical inference based on ranks. New York: Wiley. Google Scholar
- Hoeffding, W. (1952). The large sample power of tests based on permutations of observations. Annals of Mathematical Statistics, 23, 169–192. ArticleGoogle Scholar
- Hothorn, T., Hornik, K., van de Wiel, M.A., & Zeileis, A. (2006). A Lego system for conditional inference. The American Statistician, 60(3), 257–263. ArticleGoogle Scholar
- Keller-McNulty, S., & Higgins, J.J. (1987). Effect of tail weight and outliers on power and type-I error of robust permutation tests for location. Communications in Statistics. Simulation and Computation, 16, 17–35. ArticleGoogle Scholar
- Kempthorne, O., & Doerfler, T.E. (1969). The behavior of some significance tests under experimental randomization. Biometrika, 56, 231–248. ArticleGoogle Scholar
- Keppel, G., & Wickens, T.D. (2004). Design and analysis: a researcher’s handbook (4th ed.). Upper Saddle River: Pearson Education. Google Scholar
- Klotz, J.H. (1966). The Wilcoxon, ties, and the computer. Journal of the American Statistical Association, 61, 772–787. ArticleGoogle Scholar
- Lehmann, E.L. (1975). Nonparametrics. San Francisco: Holden-Day. Google Scholar
- Levin, J.R., Marascuilo, L.A., & Hubert, L.J. (1978). N=nonparametric randomization tests. In T.R. Kratochwill (Ed.), Single-subject research: strategies for evaluating change (pp. 167–196). New York: Academic Press. Google Scholar
- Ludbrook, J., & Dudley, H. (1998). Why permutation tests are superior to t and F tests in biomedical research. The American Statistician, 52, 127–132. ArticleGoogle Scholar
- Mann, H.B., & Whitney, D.R. (1947). On a test of whether one of two random variables is stochastically larger than the other. Annals of Mathematical Statistics, 18, 50–60. ArticleGoogle Scholar
- Mehta, C.R., Patel, N.R., & Tsiatis, A.A. (1984). Exact significance testing to establish treatment equivalence with ordered categorical data. Biometrics, 40, 819–825. ArticlePubMedGoogle Scholar
- Mewhort, D.J.K. (2005). A comparison of the randomization test with the F test when error is skewed. Behavior Research Methods, 37, 426–435. ArticlePubMedGoogle Scholar
- Onghena, P., & May, R.B. (1995). Pitfalls in computing and interpreting randomization test p values: A commentary on Chen and Dunlap. Behavior Research Methods, Instruments, & Computers, 27, 408–411. ArticleGoogle Scholar
- Pitman, E.J.G. (1937). Significance tests which may be applied to samples from any populations. Supplement to the Journal of the Royal Statistical Society, 4, 119–130. ArticleGoogle Scholar
- R Development Core Team (2011). R: a language and environment for statistical computing [Computer software manual]. Vienna, Austria. Available from http://www.R-project.org/ (ISBN 3-900051-07-0).
- Scheffé, H. (1959). The analysis of variance. New York: Wiley. Google Scholar
- Streitberg, B., & Röhmel, J. (1986). Exact distributions for permutation and rank tests: An introduction to some recently published algorithms. Statistical Software Newsletter, 12, 10–17. Google Scholar
- Tomarken, A.J., & Serlin, R.C. (1986). Comparison of ANOVA alternatives under variance heterogeneity and specific noncentrality structures. Psychological Bulletin, 99, 90–99. ArticleGoogle Scholar
- Toothaker, L.E. (1972). An empirical investigation of the permutationt-test as compared to Student’st-test and the Mann-WhitneyU-test. Doctoral dissertation, University of Wisconsin, Madison.
- van den Brink, W.P., & van den Brink, S.G.J. (1989). A comparison of the power of the t test, Wilcoxon’s test, and the approximate permutation test for the two-sample location problem. British Journal of Mathematical & Statistical Psychology, 42, 183–189. ArticleGoogle Scholar
- Wald, A., & Wolfowitz, J. (1944). Statistical tests based on the permutations of the observations. Annals of Mathematical Statistics, 15, 358–372. ArticleGoogle Scholar
- Wilcoxon, F. (1945). Individual comparisons by ranking methods. Biometrics, 1, 80–83. ArticleGoogle Scholar
- Zimmerman, D., & Zumbo, B. (1992). Parametric alternatives to the Student t test under violation of normality and homogeneity of variance. Perceptual and Motor Skills, 74, 835–844. Google Scholar
- Zimmerman, D., & Zumbo, B. (1993). Rank transformations and the power of the Student t test and Welch t′ test for non-normal populations with unequal variances. Canadian Journal of Experimental Psychology, 47, 523–539. ArticleGoogle Scholar
Acknowledgements
The author is tremendously grateful to Professors Jee-Seon Kim, Ronald Serlin, and Peter Steiner for helpful discussions.